Blog articles
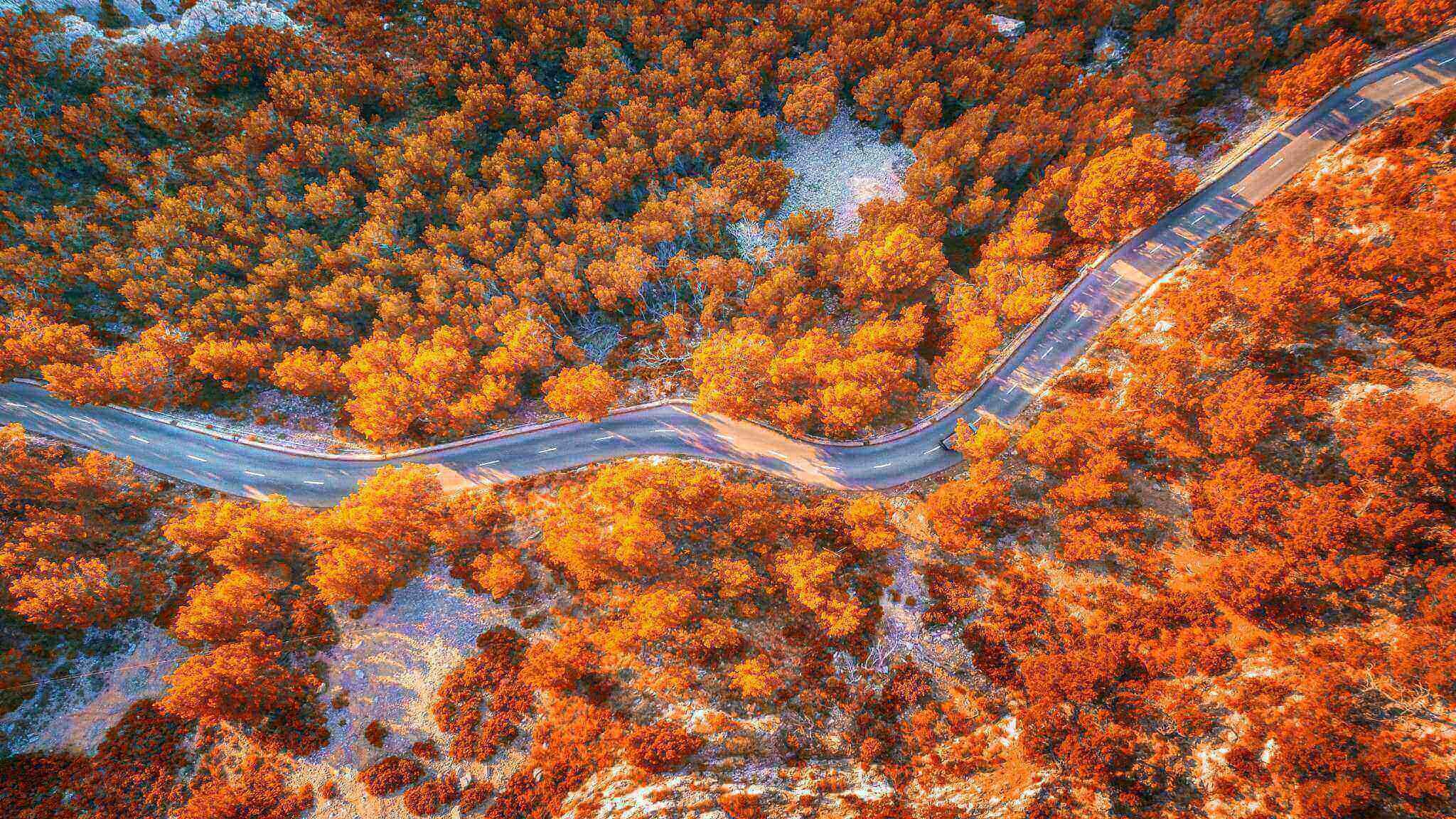
What is Microsoft Entra?
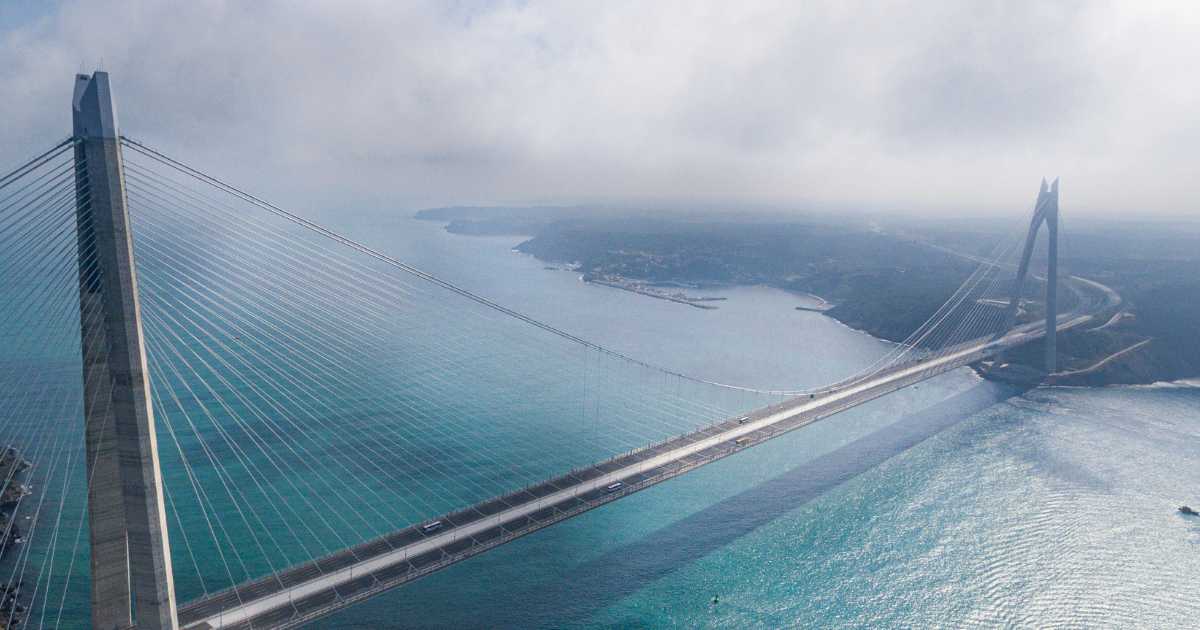
What is FinOps and how is it transforming cloud computing?
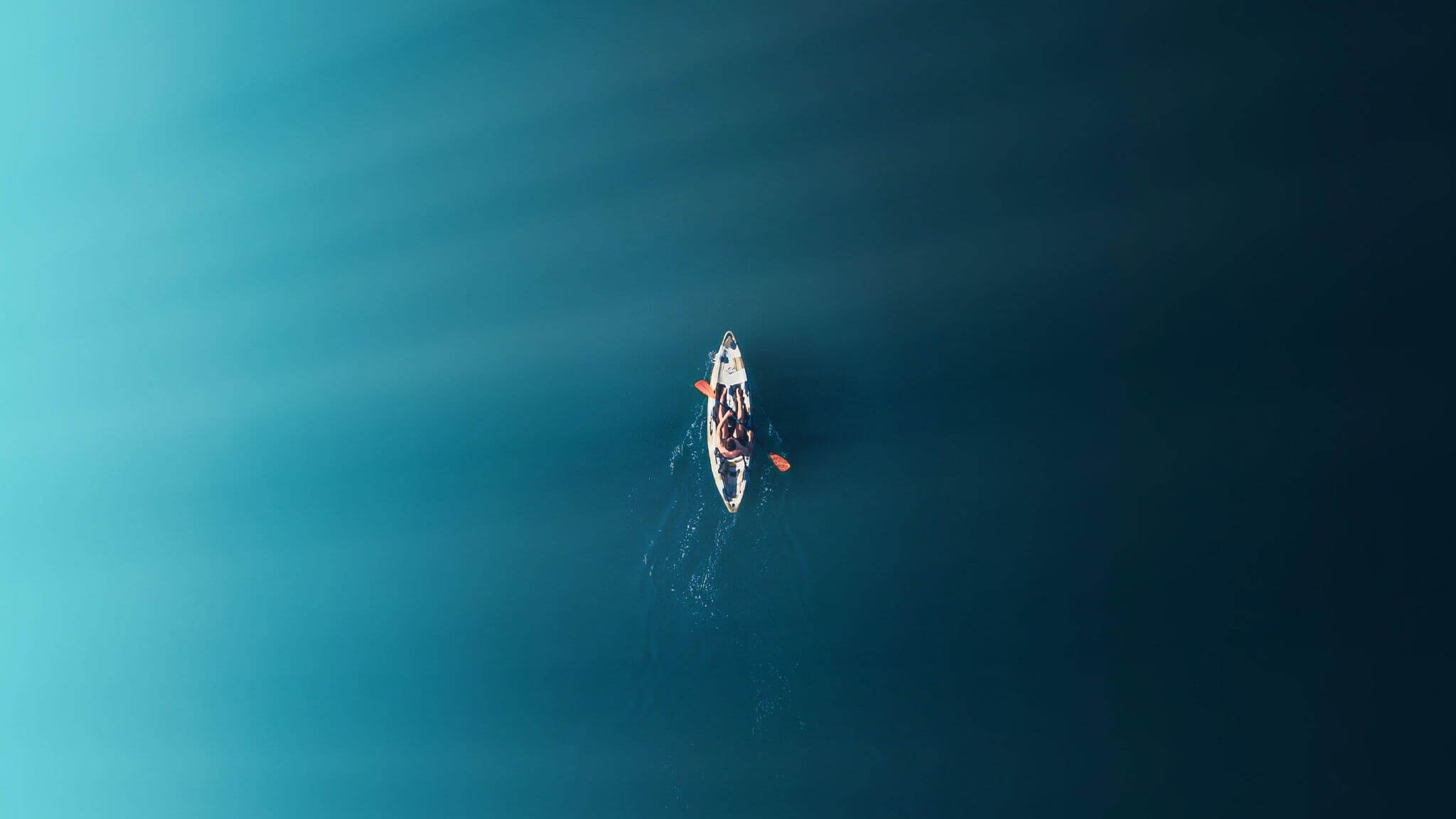
Everything you need to know about Microsoft Zero Trust Security
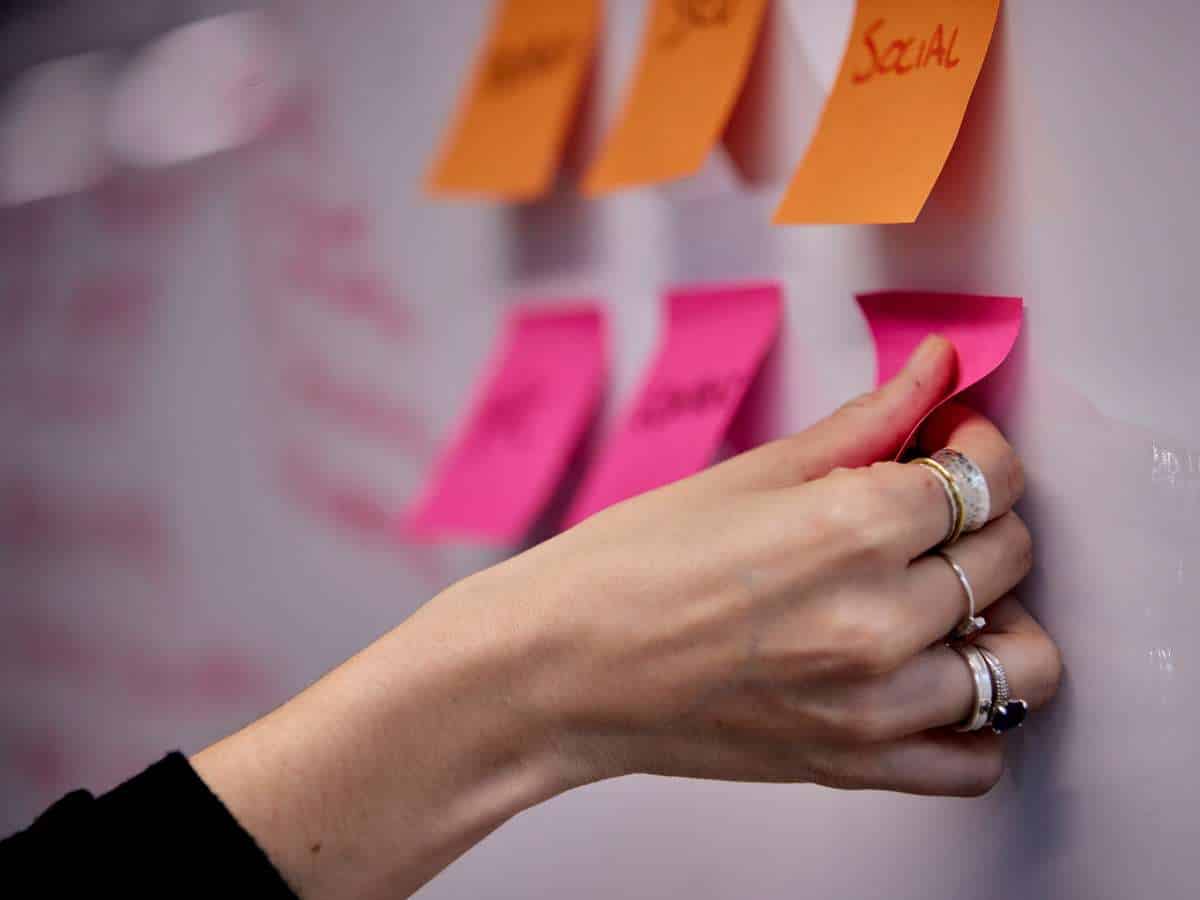
The expert’s guide to improving identity and access security
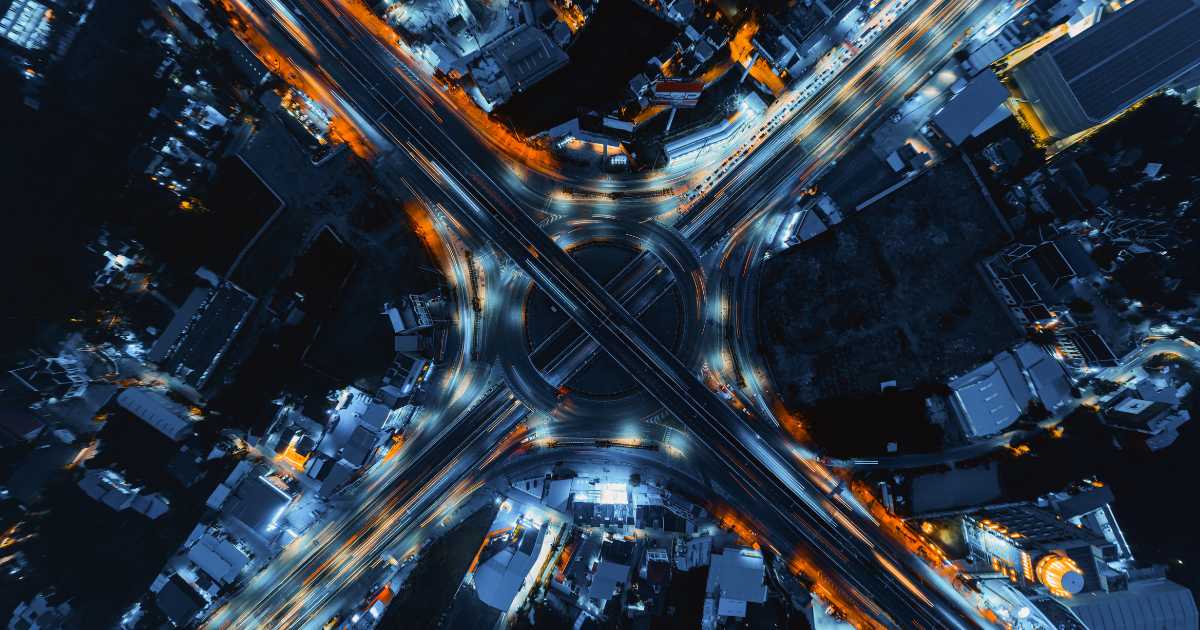
What is Microsoft Defender for Endpoint?
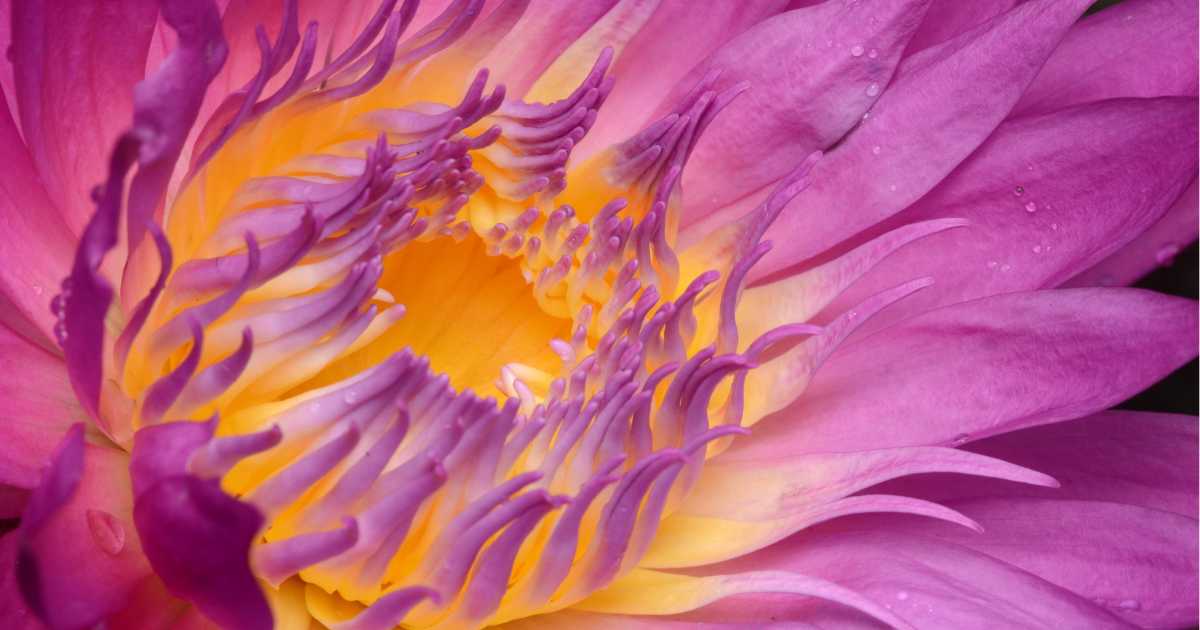
Microsoft Intune: Unlock the benefits of your BYOD strategy
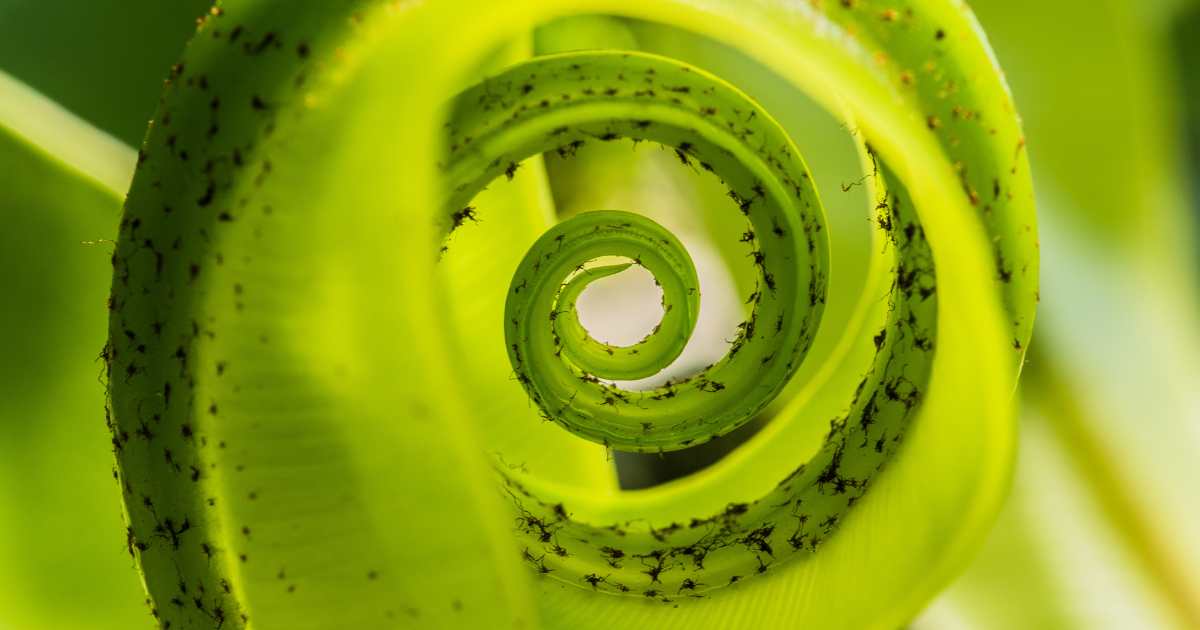
Entra ID vs Okta: Choosing the right cloud-identity solution
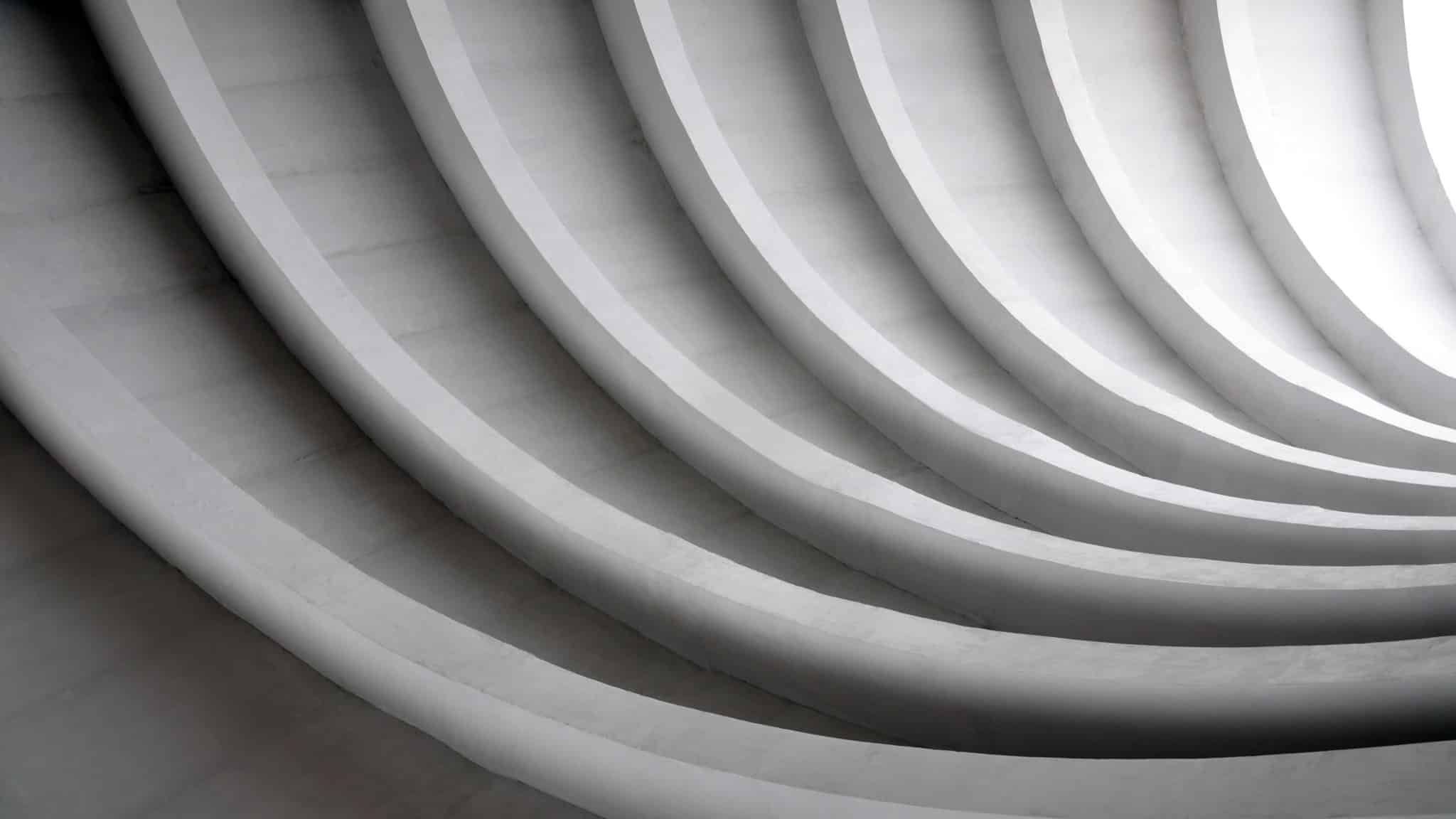
What is Microsoft Intune?
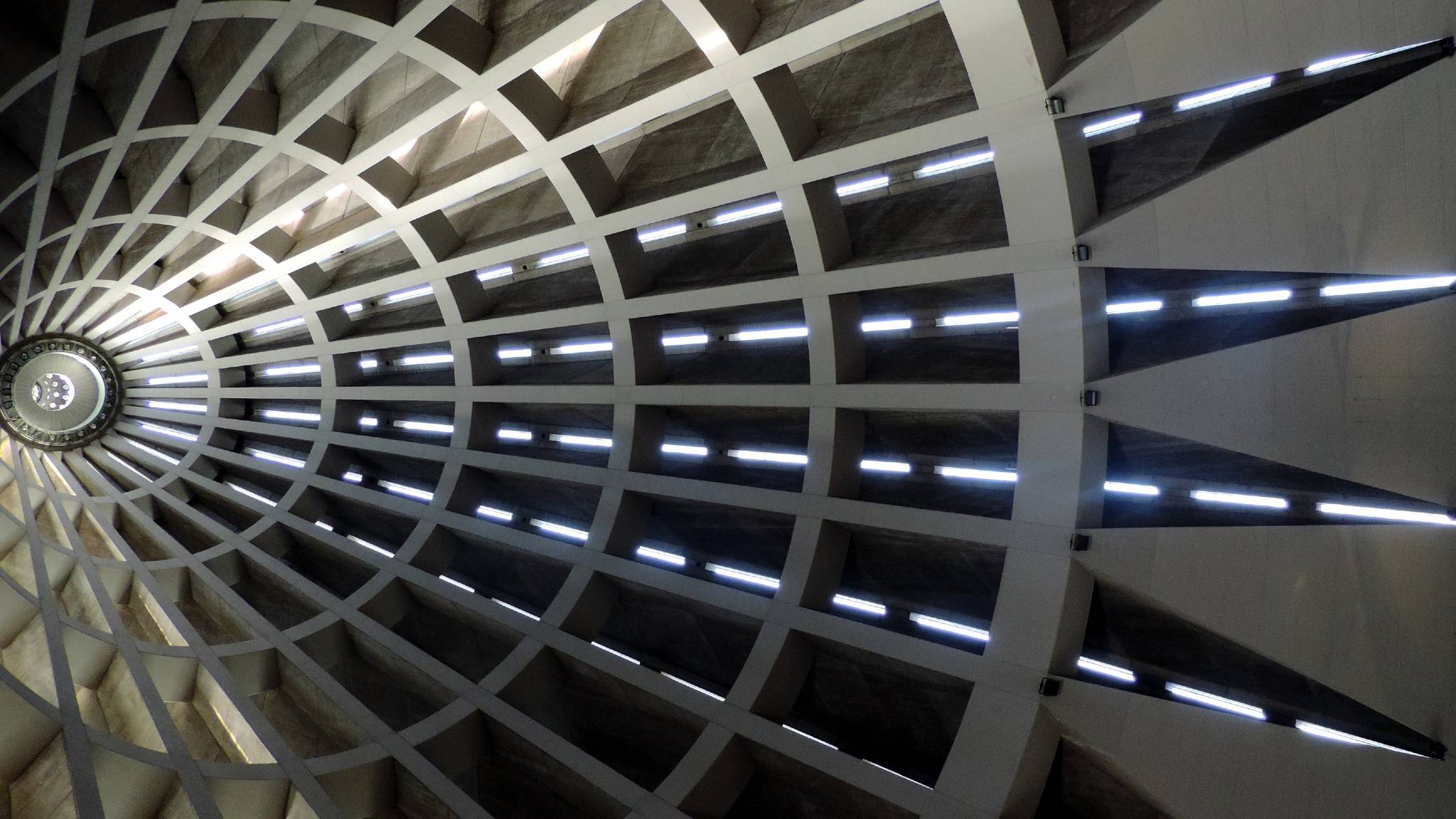
Third-party access management: Why you need to care about collaborator controls
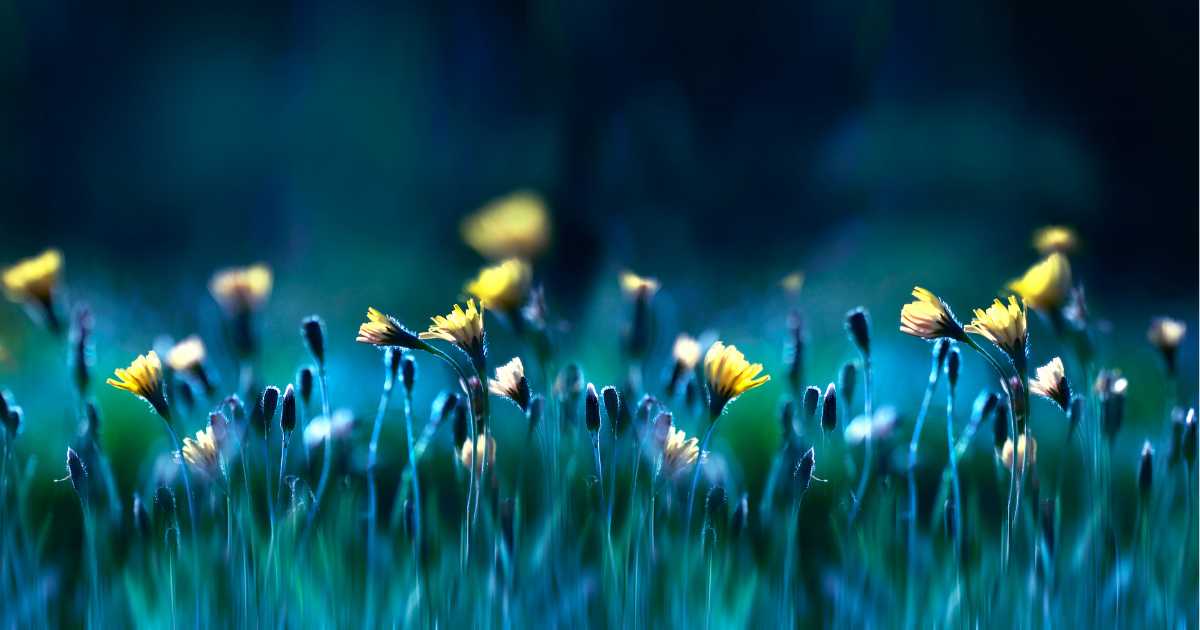
Cyber security trends for 2024
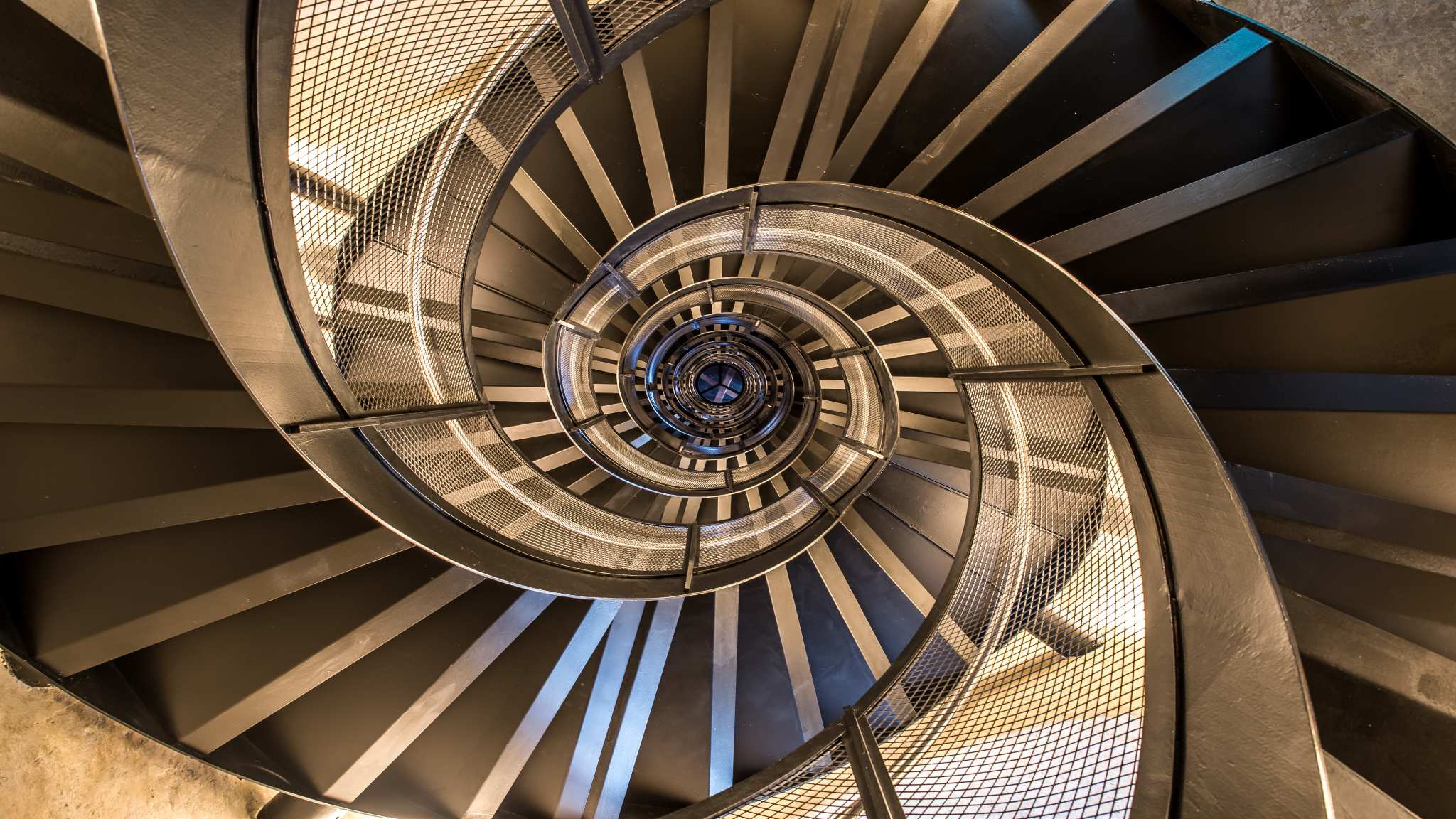
Improving identity lifecycle management with lifecycle workflows
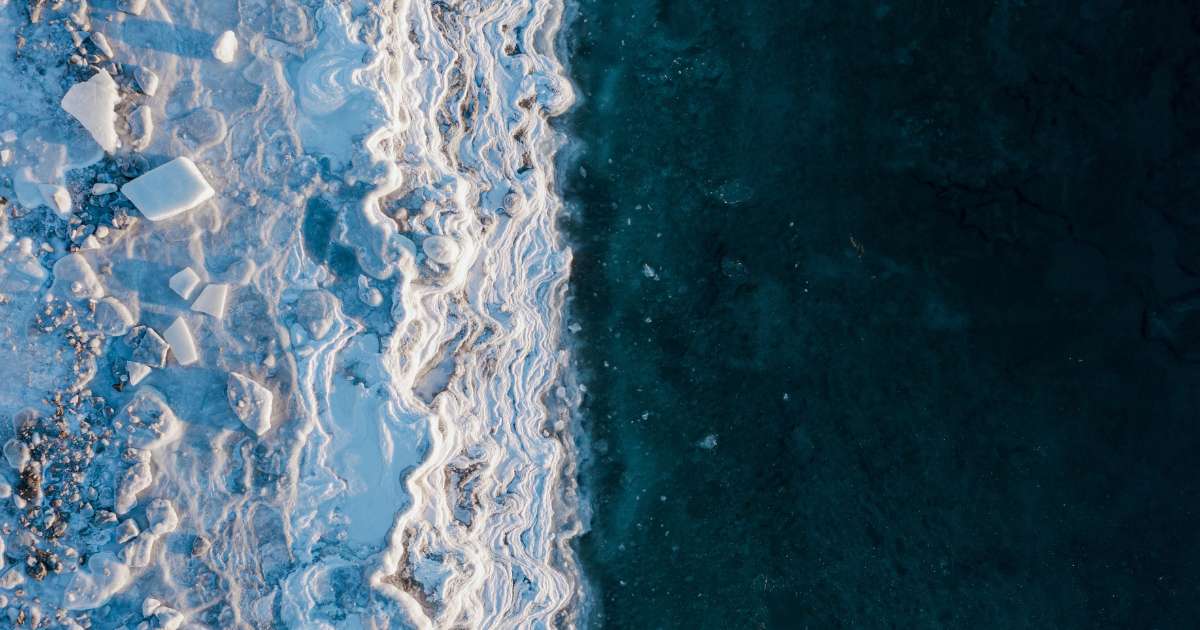
5 privileged access management (PAM) best practices
Great emails start here
Sign up for free resources and exclusive invites
Subscribe to the Kocho mailing list if you want:
- Demos of the latest Microsoft tech
- Invites to exclusive events and webinars
- Resources that make your job easier
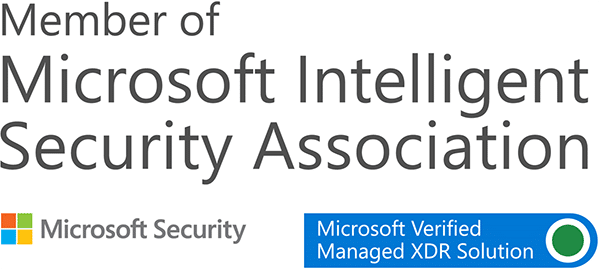
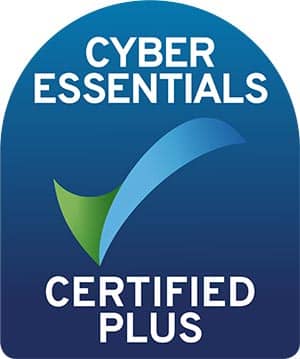
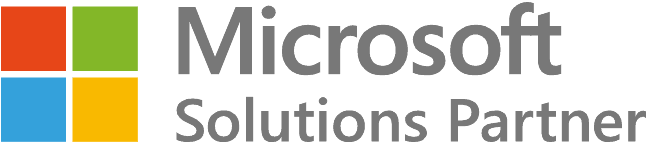

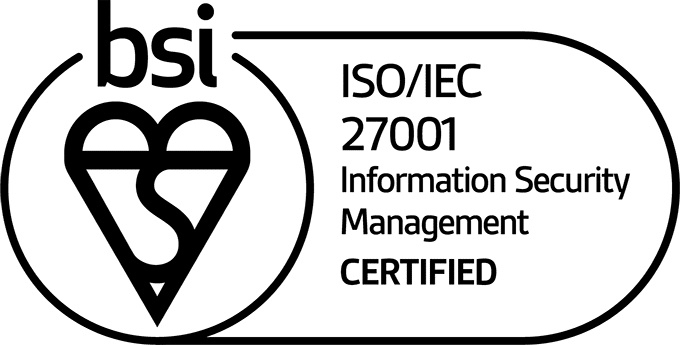
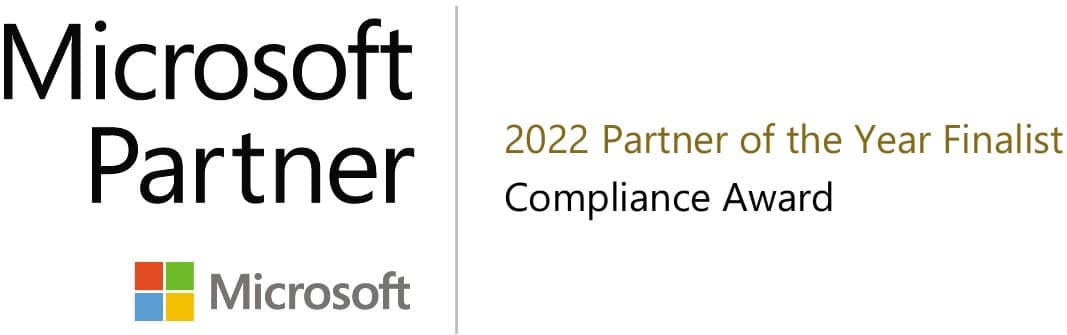
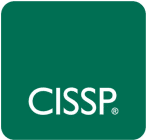
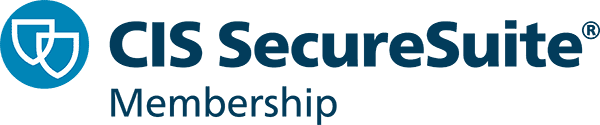
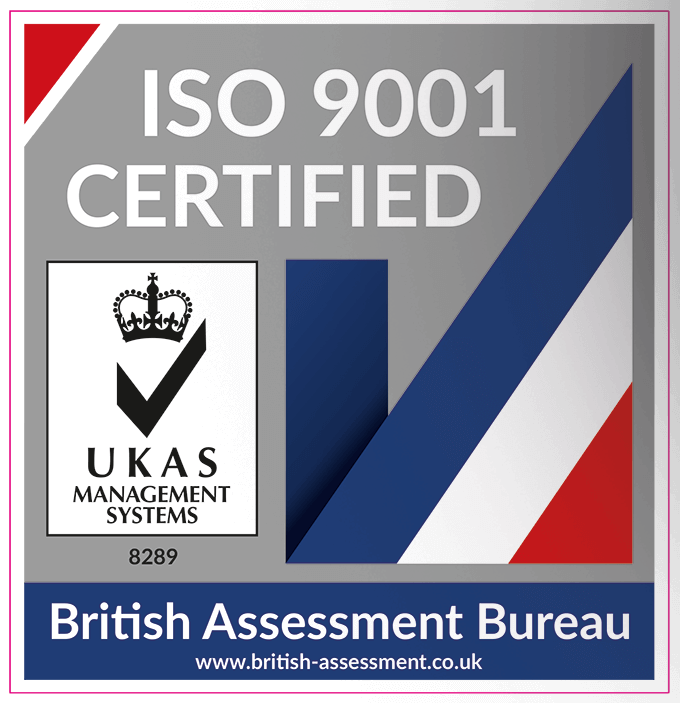
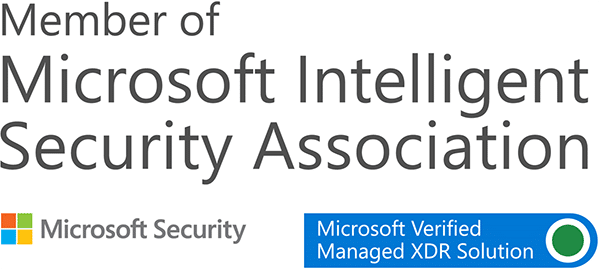
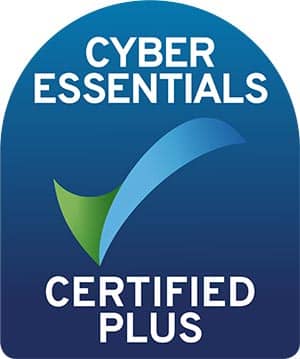
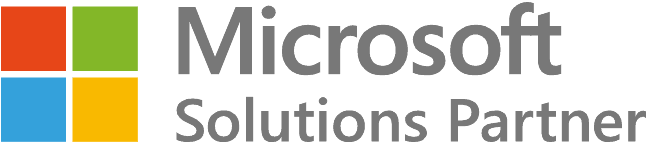

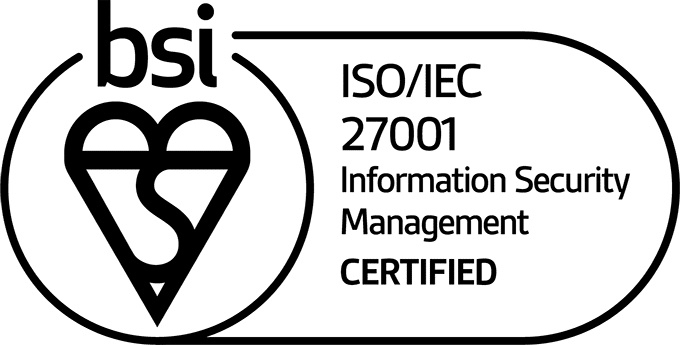
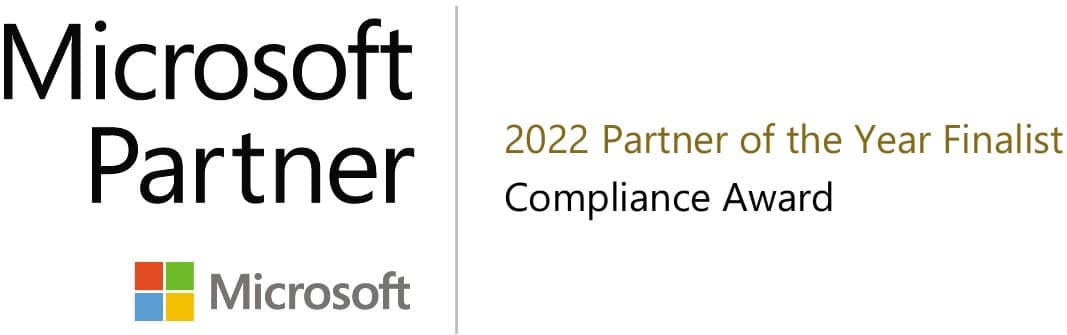
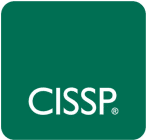
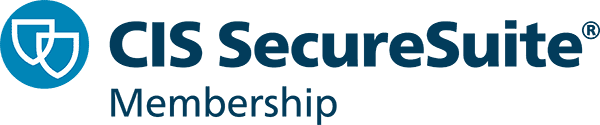
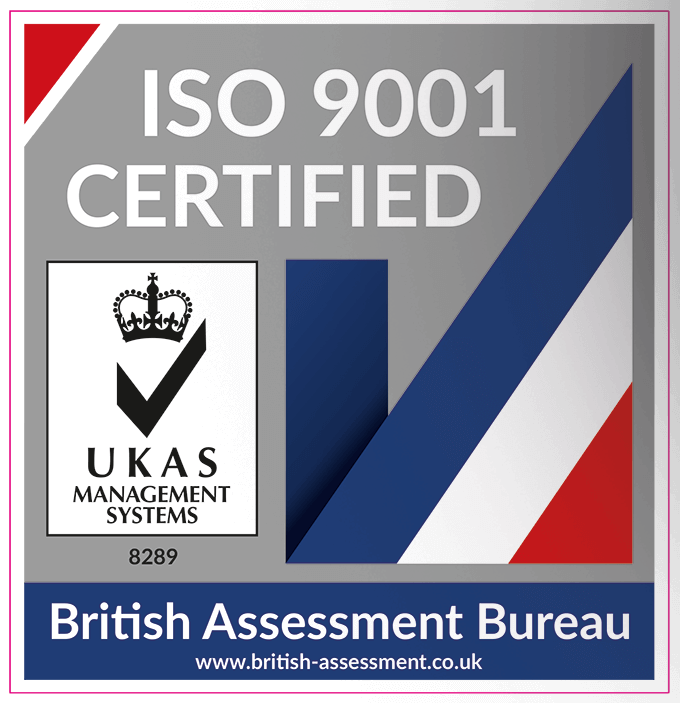
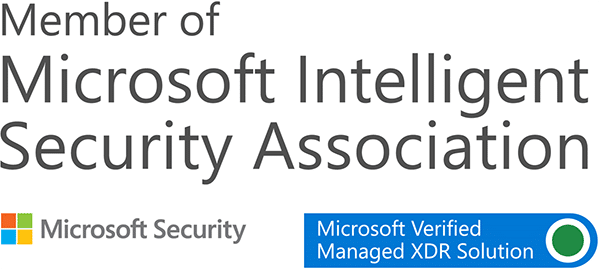
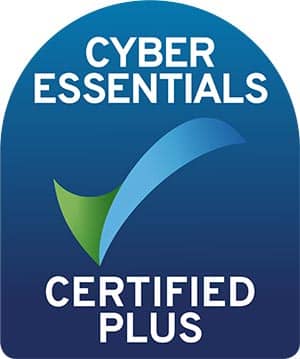
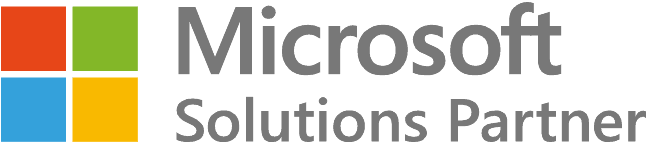

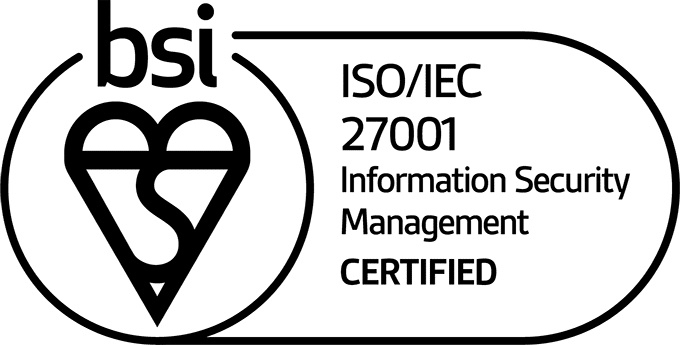
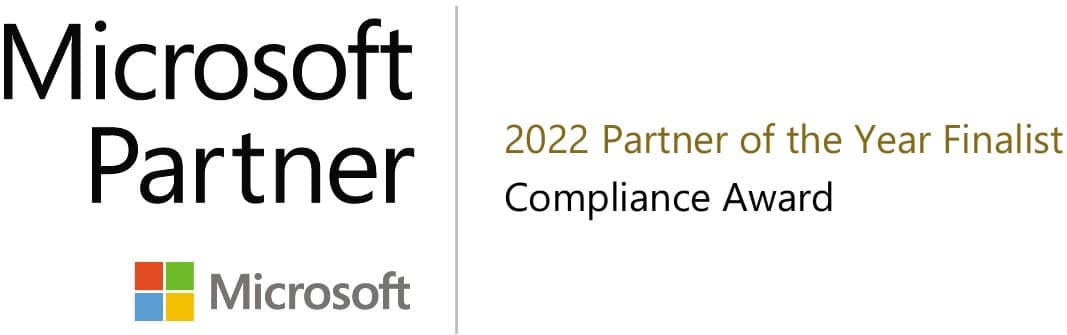
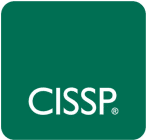
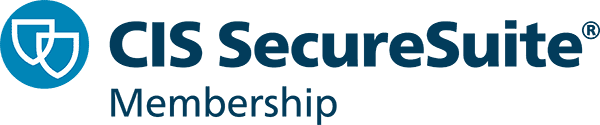
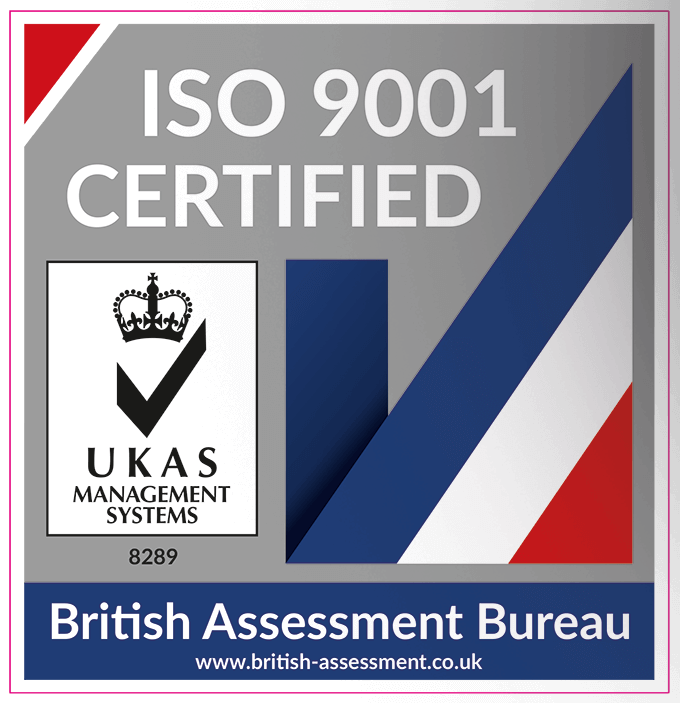
Got a question? Need more information?
Our expert team is here to help.